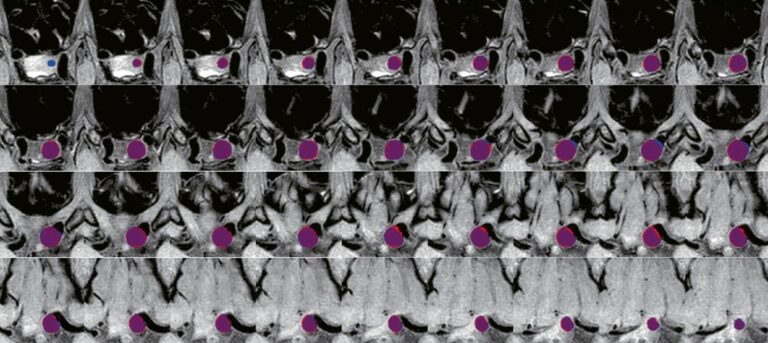
A deep learning framework for intracranial aneurysms automatic segmentation and detection on magnetic resonance T1 images
This study featured a design of a deep learning-based framework for the automatic segmentation of intracranial aneurysms (IAs) on MR T1 images while also testing the robustness and performance of the framework. The authors were able to conclude that their deep learning framework could effectively detect and segment IAs using clinical routine T1 sequences, which offers potential in improving the